AWS, Goldman Sachs examine block-encoding for loading classical data
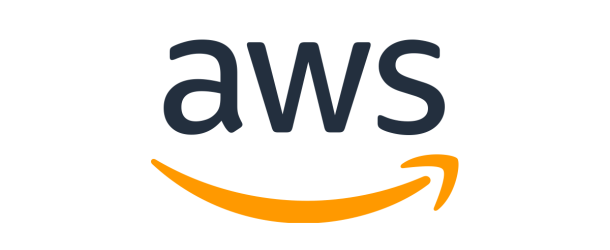
The Amazon Quantum Solutions Lab (QSL) and the AWS Center for Quantum Computing (CQC) teamed up with Goldman Sachs R&D team on a project to advance how classical data can be block-encoded into quantum memory, which could have major implications for how classical data gets loaded into quantum processors for computation.
Block-encoding makes use of special purpose data structures, such as a quantum version of a random access memory, or QRAM, to load the data, and the project partners tasked themselves with trying to find out what resources would be need to make block encoding work and how practical cost assessments could be carried out.
The process is discussed in detail in this AWS blog post, and a related technical paper, but–spoiler alert–the blog post concludes, “…we have shown that the number of qubits needed to load classical data using traditional methods of block-encoding might be prohibitively expensive without major advancements in quantum computing technology. However, our results also show that we can achieve circuit depths that are only logarithmic in the size of the classical dataset, which suggests that quantum algorithms relying on block-encoding could be extremely efficient if we have access to extremely large numbers of QRAM qubits (i.e., a number that has to scale with the size of the input data).”
AWS operates the Amazon Braket cloud-based quantum computing service, and earlier this year launched a Center for Quantum Networking to go along with its QSL and CQC initiatives. Goldman Sachs, meanwhile, has worked on a number of quantum computing projects, working with QCware, IonQ, and others.
Dan O’Shea has covered telecommunications and related topics including semiconductors, sensors, retail systems, digital payments and quantum computing/technology for over 25 years.