(ZDNet) Machine learning is a well-established branch of artificial intelligence that is already used in many industries to solve a variety of business problems. The approach consists of training an algorithm with large datasets, to enable the model to identify different patterns and eventually calculate the best answer when presented with new information.
One method in particular, called quantum kernels, is the focus of many research papers. In the quantum kernel approach, the quantum computer steps in for only one part of the overall algorithm, by expanding what is known as the feature space – the collection of features that are used to characterize the data that is fed to the model, such as “gender” or “age”, if the system is trained to recognize patterns about people.
IBM’s researchers set out to use quantum kernels to solve a specific type of machine-learning problem called classification. As IBM’s team explains, the most standard example of a classification problem is when a computer is given pictures of dogs and cats, and is required to train with this dataset to label all future images it sees as either a dog or a cat, with the goal of generating accurate labels in as little time as possible.
Big Blue’s scientists developed a new classification task and found that a quantum algorithm using the quantum kernel method is capable of finding relevant features in the data for accurate labeling, while for classical computers the dataset looked like random noise.
The researchers created a classification problem for which the data can be generated on a classical computer, and showed that no classical algorithm can do better than random guessing when attempting to solve the problem.
“This paper can be viewed as a milestone in the field of quantum machine learning, since it proves an end-to-end quantum speed-up for a quantum kernel method implemented fault-tolerantly with realistic assumptions,” concluded the research team.
IBM Shows Quantum Computer Can Solve Problems Classical Computers Find Hard
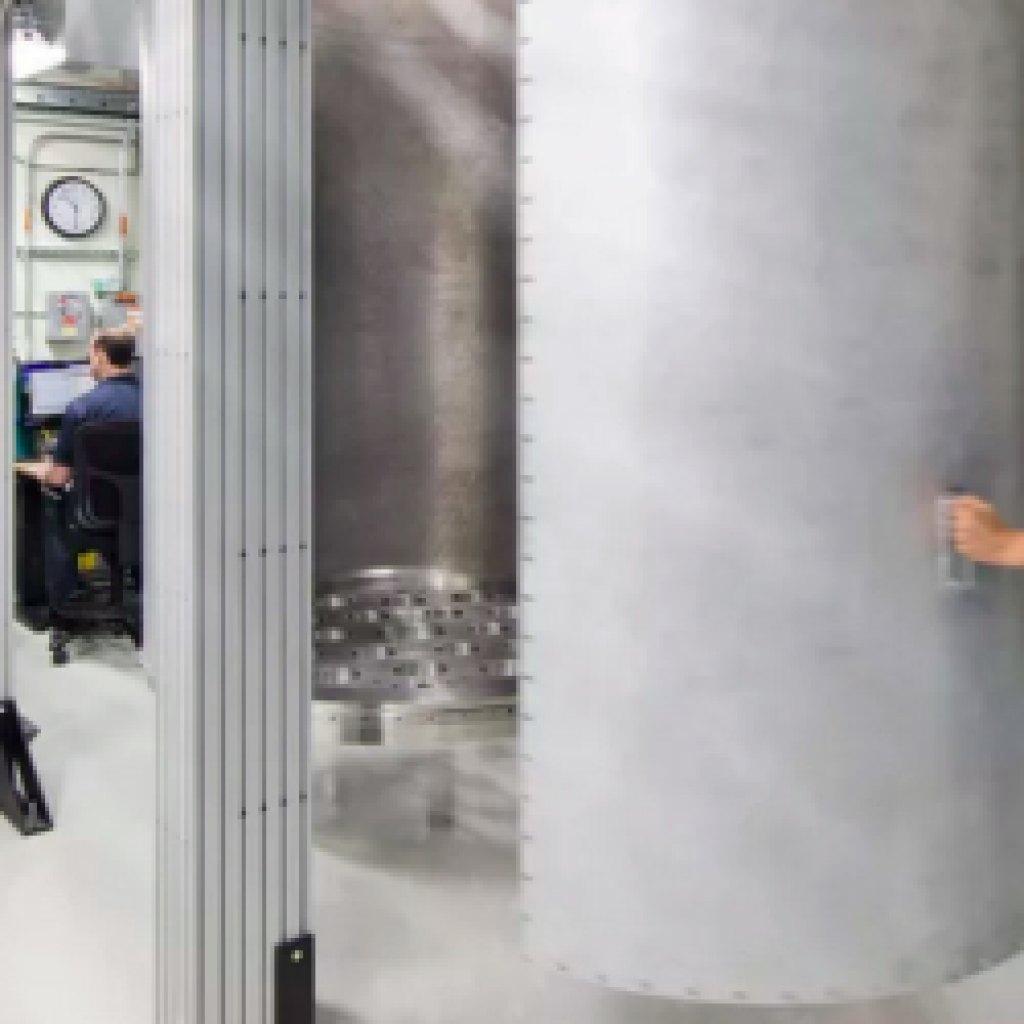