(HPCWire) Computer scientists led by ETH Zurich have conducted an early exploration for reliable quantum machine learning.
With very large and complex data sets, machine learning can deliver valuable results that humans would not be able to find out, or only with much more time. However, for certain computational tasks, even the fastest computers available today reach their limits. This is where the great promise of quantum computers comes into play: that one day they will also perform super-fast calculations that classical computers cannot solve in a useful period of time.
The reason for this “quantum supremacy” lies in physics: quantum computers calculate and process information by exploiting certain states and interactions that occur within atoms or molecules or between elementary particles.
The fact that quantum states can superpose and entangle creates a basis that allows quantum computers the access to a fundamentally richer set of processing logic.
These speed advantages of quantum computing are also an opportunity for machine learning applications – after all, quantum computers could compute the huge amounts of data that machine learning methods need to improve the accuracy of their results much faster than classical computers.
A reliable quantum classification algorithm correctly classifies a toxic mushroom as “poisonous” while a noisy, perturbed one classifies it faultily as “edible”.
However, to really exploit the potential of quantum computing, one has to adapt the classical machine learning methods to the peculiarities of quantum computers. For example, the algorithms, i.e. the mathematical calculation rules that describe how a classical computer solves a certain problem, must be formulated differently for quantum computers. Developing well-functioning “quantum algorithms” for machine learning is not entirely trivial, because there are still a few hurdles to overcome along the way
The research group led by Ce Zhang, ETH computer science professor and member of the ETH AI Center, has has recently explored, somehow “accidentally” during an endeavor to reason about the robustness of classical distributions for the purpose of building better machine learning systems and platforms. Together with Professor Nana Liu from Shanghai Jiao Tong University and with Professor Bo Li from the University of Illinois at Urbana, they have developed a new approach. This allows them to prove the robustness conditions of certain quantum-based machine learning models, for which the quantum computation is guaranteed to be reliable and the result to be correct. The researchers have published their approach, which is one of the first of its kind, in the scientific journal “npj Quantum Information”.
Computer Scientists Led by ETH Zurich Explore Reliable Quantum Machine Learning
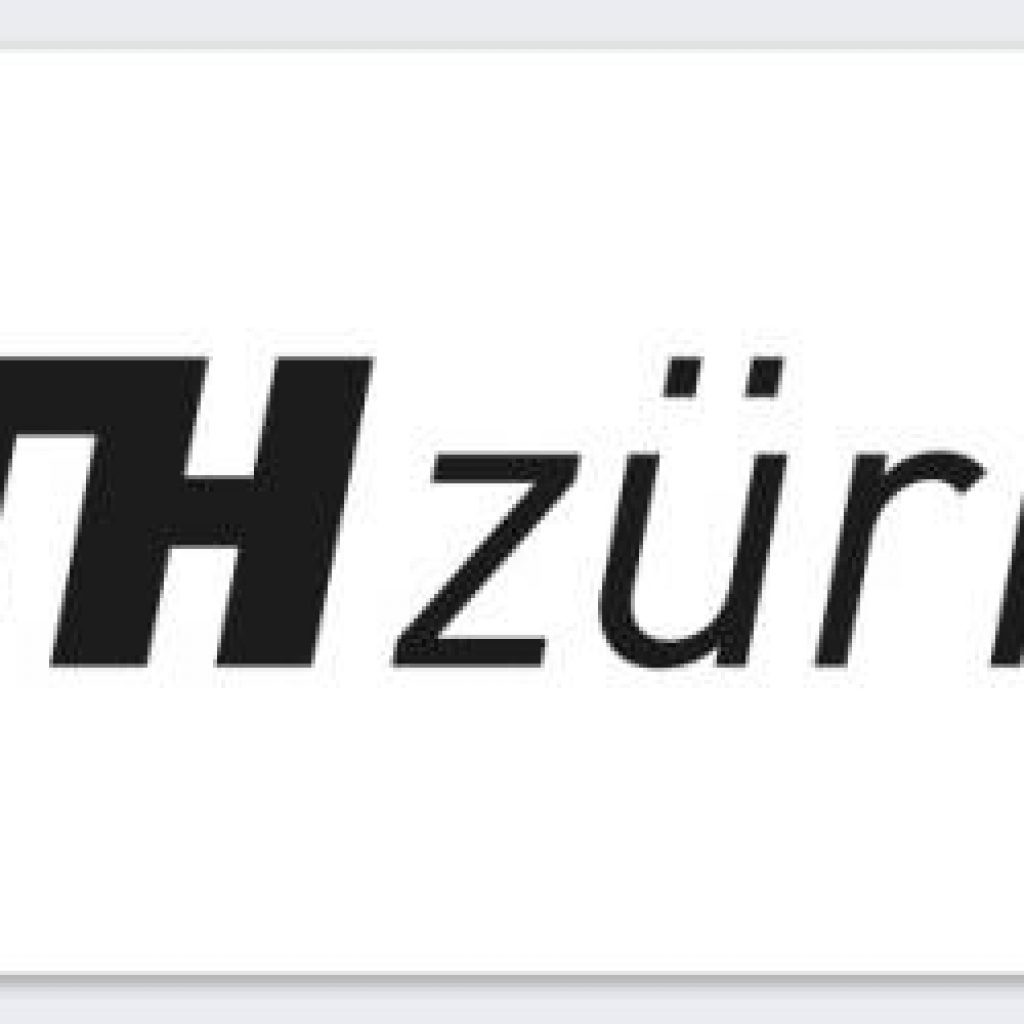