(ScienceAlert) a pair of physicists from École Polytechnique Fédérale de Lausanne (EPFL) in Switzerland and Columbia University in the US have come up with a way to judge the potential of near-term quantum devices – by simulating the quantum mechanics they rely upon on more traditional hardware.
Their study made use of a neural network developed by EPFL’s Giuseppe Carleo and his colleague Matthias Troyer back in 2016, using machine learning to come up with an approximation of a quantum system tasked with running a specific process.
Known as the Quantum Approximate Optimization Algorithm (QAOA), the process identifies optimal solutions to a problem on energy states from a list of possibilities, solutions that should produce the fewest errors when applied.
“There is a lot of interest in understanding what problems can be solved efficiently by a quantum computer, and QAOA is one of the more prominent candidates,” says Carleo.
The QAOA simulation developed by Carleo and Matija Medvidović, a graduate student from Columbia University, mimicked a 54 qubit device. While it was an approximation of how the algorithm would run on an actual quantum computer, it did a good enough job to serve as the real deal.Time will tell if physicists of the future will be quickly crunching out ground states in an afternoon of QAOA calculations on a bona fide machine, or take their time using tried-and-true binary code.
“But the barrier of ‘quantum speedup’ is all but rigid and it is being continuously reshaped by new research, also thanks to the progress in the development of more efficient classical algorithms,” says Carleo.
As tempting as it might be to use simulations as a way to argue classical computing retains an advantage over quantum machines, Carleo and Medvidović insist the approximation’s ultimate benefit is to establish benchmarks in what could be achieved in the current era of newly emerging, imperfect quantum technologies.
Scientists Simulated Quantum Technology on Classical Computing Hardware
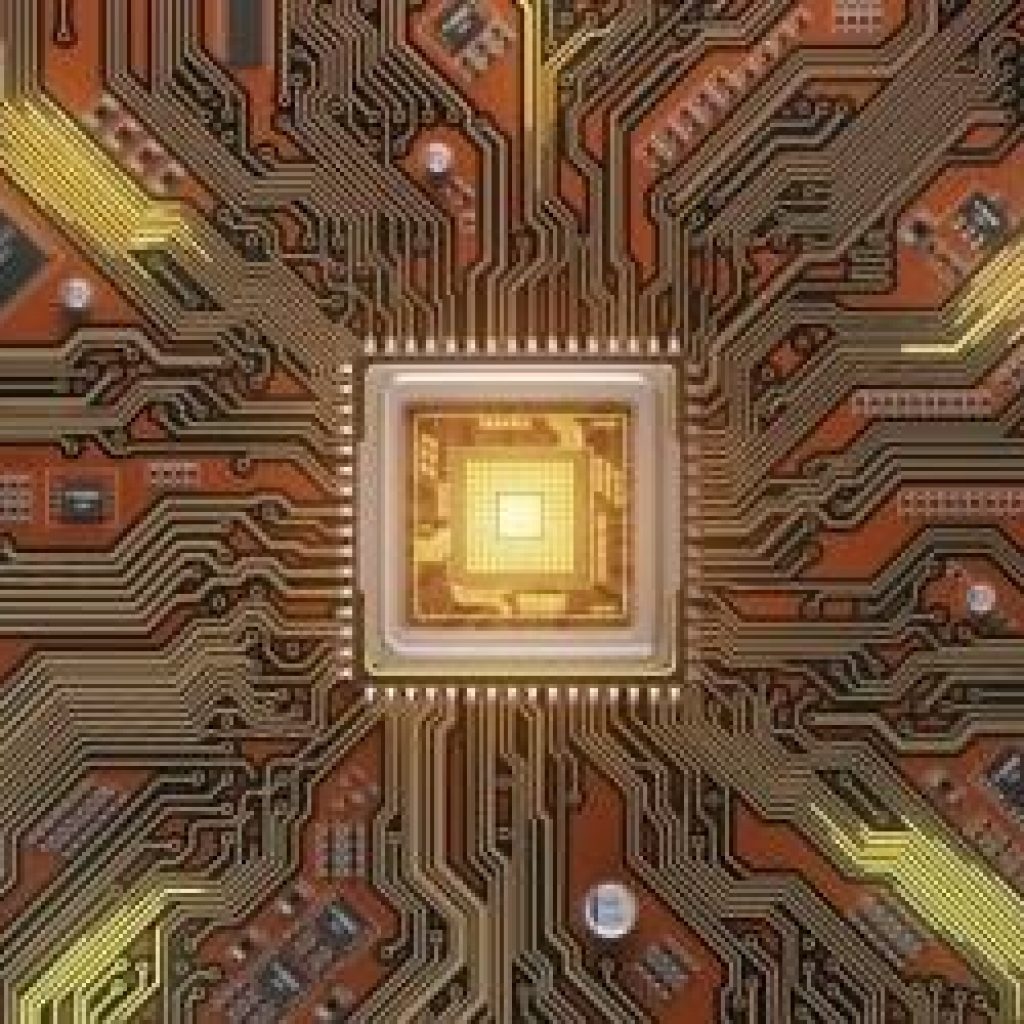